AI for Predictive Functional Outcomes & Prognosis in Orthopaedic & Personal Injury Cases
- Dr Suhail Chughtai FRCS
- Dec 14, 2024
- 4 min read
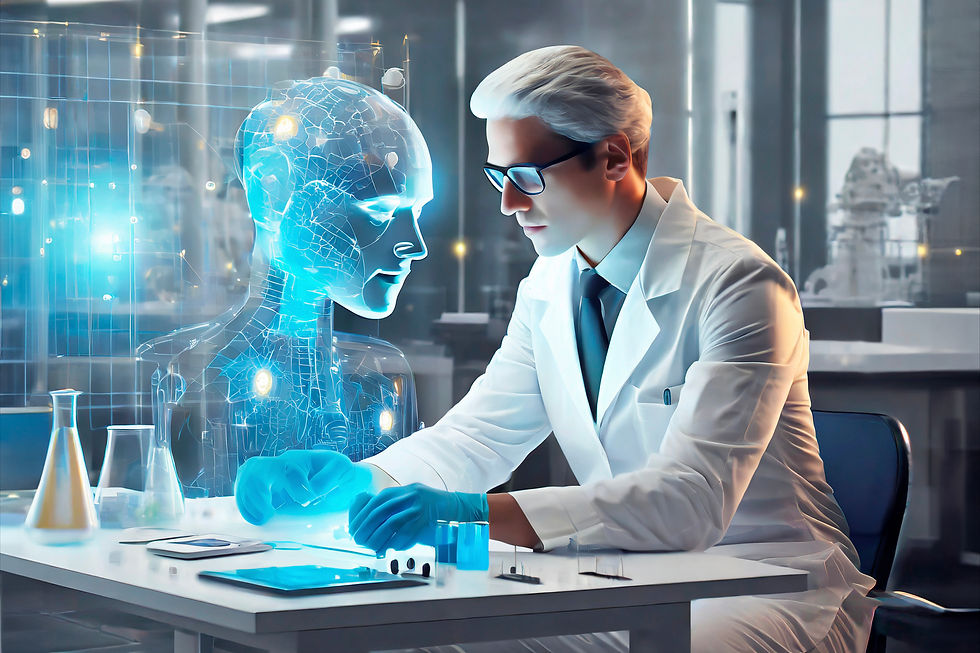
Blog by: Dr. Suhail Chughtai, FRCS, FFLM
Introduction
Artificial Intelligence (AI) and machine learning (ML) are transforming various aspects of healthcare, with orthopaedics and personal injury cases being no exception. By leveraging data from injury types, treatment plans, and patient demographics, AI models offer an innovative approach to predicting long-term functional outcomes and rehabilitation needs. These models are becoming increasingly significant in medico-legal contexts, assisting clinicians and legal professionals in making informed decisions (Johansson et al., 2020).
Machine Learning in Predictive Functional Outcomes
AI-driven predictive models have gained prominence in orthopaedic injuries. These models analyze a wealth of patient data, including injury type, patient demographics, and treatment regimens, to forecast recovery trajectories. Machine learning algorithms such as decision trees, random forests, and neural networks are widely employed in predicting post-operative complications, recovery time, and long-term outcomes, including pain levels and mobility. For example, Johansson et al. (2020) demonstrated how machine learning can predict recovery after orthopaedic surgery with significant accuracy, helping clinicians tailor rehabilitation strategies and providing legal professionals with reliable predictions for future care needs.
A relevant case study from Li et al. (2021) explored the application of machine learning in predicting functional recovery for elderly patients with hip fractures. By analyzing factors like fracture type and treatment approach, the model achieved an accuracy of 85% in predicting mobility outcomes six months post-surgery. Such predictive capabilities are critical in medico-legal cases, where long-term care costs and rehabilitation needs are often central to legal settlements (Li et al., 2021).
Role in Medico-Legal Decisions
The ability to predict long-term outcomes with AI significantly impacts personal injury claims and medico-legal cases. Legal proceedings often hinge on expert opinions regarding a patient’s recovery, rehabilitation needs, and the potential for permanent disability. AI’s role is crucial here, as it can provide consistent, evidence-based predictions that reduce the subjectivity of expert testimony. In medico-legal settings, AI’s accuracy in forecasting long-term outcomes can assist in calculating rehabilitation costs, estimating future medical needs, and assessing the likelihood of permanent impairment. This helps in ensuring fairer and more equitable compensation, especially in cases involving complex injuries or vulnerable populations like the elderly or individuals with pre-existing conditions (Keegan et al., 2022).
Deployment Methodology
Deploying AI for predictive functional outcomes in orthopaedics requires several key steps. First, high-quality, comprehensive datasets are gathered, which include clinical outcomes, patient demographics, and rehabilitation progress. Data preprocessing is essential to handle missing or inconsistent information, which can impact model accuracy. The machine learning models are then trained using historical data to identify patterns linking various factors—such as injury type, demographics, and treatment plans—to specific outcomes. Algorithms like random forests, neural networks, and support vector machines are commonly used for this task. Once trained, the models can provide real-time predictions, offering valuable insights that clinicians can use to tailor rehabilitation plans and legal professionals can use for estimating long-term care needs (Johansson et al., 2020).
Challenges in Deployment
Despite its promise, several challenges hinder the widespread adoption of AI in this field. The quality and availability of data are key limitations, as large, diverse datasets are necessary to train accurate models. Additionally, ensuring model interpretability is a significant hurdle; AI systems, particularly deep learning models, are often criticized for being "black boxes" where it’s difficult to understand how predictions are made. The integration of AI tools into clinical workflows also poses challenges, particularly in ensuring that these tools align with existing electronic health record (EHR) systems. Ethical concerns, such as data privacy and patient consent, are another critical consideration, particularly in medico-legal contexts where sensitive health data is involved (Keegan et al., 2022).
Future Vision
Looking ahead, AI’s role in orthopaedics and personal injury cases is expected to grow significantly. Advancements in wearable technology and real-time data collection will improve the accuracy of predictive models, allowing for more personalized and dynamic treatment plans. Additionally, the integration of multimodal data—combining clinical records with imaging, genetic information, and patient-reported outcomes—will refine predictions and offer a more comprehensive understanding of patient prognoses. AI will also continue to evolve with continuous learning capabilities, meaning predictions will improve as treatment methods advance, ensuring that models remain current and reliable. In the future, these AI-driven tools will become an essential part of both clinical decision-making and medico-legal processes, offering more precise and equitable outcomes for all involved (Li et al., 2021).
Conclusion
AI offers a transformative potential for predicting long-term functional outcomes and rehabilitation needs in orthopaedic injuries. By improving the accuracy and consistency of predictions, AI models are revolutionizing not only patient care but also the way medico-legal cases are handled. While challenges related to data quality, interpretability, and integration remain, the future of AI in orthopaedics looks promising, with greater accuracy, personalization, and integration into clinical and legal workflows on the horizon (Zhang et al., 2023).
..........................................................................................................
DISCLAIMER
The content presented in this publication includes references, insights, and excerpts derived from external sources and authors. Every effort has been made to credit the original authors and sources appropriately. If any oversight or misrepresentation is identified, it is unintentional, and we welcome corrections to ensure proper attribution. The inclusion of external materials does not imply endorsement or affiliation with the original authors or publishers. This publication is intended for informational and educational purposes only, and the views expressed are those of the author(s) and do not necessarily reflect the opinions of the referenced sources.
Kommentare