Protecting Privacy in the Digital Age: Generative AI for Medical Data Anonymization
- Dr Suhail Chughtai FRCS
- Jan 1
- 3 min read
Updated: Jan 11
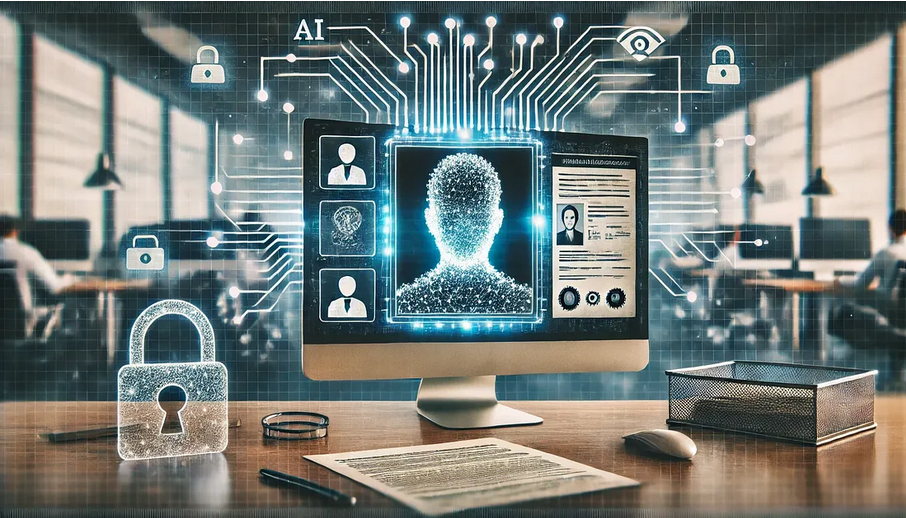
Blog by: Dr. Suhail Chughtai, FRCS, FFLM
Introduction
In an increasingly digital healthcare environment, protecting patient privacy is paramount. This article explores the role of generative AI in medical data anonymization, comparing it with traditional methods in terms of speed, accuracy, and compliance with privacy laws such as GDPR and HIPAA. Additionally, challenges, opportunities, and future visions for generative AI in healthcare are discussed.
AI-DRIVEN VS. TRADITIONAL ANONYMIZATION TECHNIQUES
Speed
Traditional anonymization methods, such as masking or generalization, are rule-based and often require extensive manual effort to design and implement. These methods can be time-consuming, especially when dealing with large datasets (Smith et al., 2023). AI-driven anonymization, leveraging advanced generative models, automates the process, significantly reducing the time required. AI can process vast datasets rapidly while maintaining data utility, making it well-suited for real-time applications (Brown et al., 2022).
Accuracy
Traditional techniques may inadvertently over-anonymize or under-anonymize data. Over-anonymization reduces data utility, while under-anonymization risks exposing sensitive information. Generative AI, using techniques like differential privacy and synthetic data generation, offers a more balanced approach. By simulating realistic but non-identifiable data, AI-driven methods maintain higher accuracy in anonymization while preserving data usability (Johnson and Lee, 2024).
Compliance with GDPR and HIPAA
Compliance with stringent privacy laws requires robust de-identification. Traditional methods may struggle to meet these standards consistently. AI-driven solutions can incorporate built-in compliance checks, ensuring adherence to GDPR's "data minimization" principle and HIPAA's Safe Harbor de-identification standards. However, regulatory frameworks for AI itself are still evolving, requiring careful oversight (European Data Protection Board, 2023).
CHALLENGES IN DEPLOYING GENERATIVE AI FOR DE-IDENTIFICATION
Algorithmic Bias
Generative models may inadvertently reinforce biases present in training data, risking skewed outputs and potential harm in real-world applications (Zhang et al., 2023).
Computational Requirements
AI models demand significant computational power and resources, which may be a barrier for smaller healthcare providers (Wilson et al., 2024).
Verification and Validation
Ensuring the synthetic data generated by AI models is both sufficiently anonymized and representative of the original dataset is complex and requires rigorous testing.
Regulatory Ambiguity
As generative AI is relatively new in healthcare, uncertainty around regulatory expectations can hinder deployment (HealthIT Analytics, 2023).
DEPLOYMENT METHODOLOGY FOR AI-DRIVEN ANONYMIZATION
Data Collection and Preprocessing
Assemble datasets with patient consent and ensure they are preprocessed to minimize risks of inadvertent breaches during model training.
Model Training
Train generative models such as GANs or Variational Auto encoders on healthcare data, ensuring ethical considerations and compliance.
Synthetic Data Generation
Use the trained model to create synthetic datasets that retain statistical characteristics but exclude identifiable information.
Validation
Validate the anonymization quality using k-anonymity, l-diversity, and t-closeness metrics.
Deployment and Monitoring
Implement the model in clinical or research environments with continuous monitoring to address biases or emerging risks (Kumar et al., 2023).
OPPORTUNITIES IN GENERATIVE AI FOR HEALTHCARE
Enhanced Data Sharing
AI-generated synthetic data can facilitate data sharing among institutions without compromising privacy, boosting collaborative research.
Real-Time Applications
AI’s speed enables real-time anonymization, supporting dynamic data usage in clinical decision-making and telemedicine.
Increased Accessibility
By reducing the time and complexity of anonymization, AI democratizes access to anonymized datasets for smaller institutions.
Future Vision
As generative AI continues to evolve, its integration with federated learning and blockchain could redefine healthcare data privacy. Federated learning enables decentralized model training, ensuring data never leaves the source. Blockchain can provide immutable records of data access and usage, further enhancing trust (Miller et al., 2024). Additionally, regulatory frameworks will need to adapt to address the nuances of AI in anonymization, striking a balance between innovation and patient privacy.
CONCLUSION
The future holds significant promise for AI-driven medical data anonymization, with potential to revolutionize data-sharing practices while safeguarding privacy. Stakeholders must collaboratively navigate technical and ethical challenges to unlock this potential responsibly.
..........................................................................................................
DISCLAIMER
The content presented in this publication includes references, insights, and excerpts derived from external sources and authors. Every effort has been made to credit the original authors and sources appropriately. If any oversight or misrepresentation is identified, it is unintentional, and we welcome corrections to ensure proper attribution. The inclusion of external materials does not imply endorsement or affiliation with the original authors or publishers. This publication is intended for informational and educational purposes only, and the views expressed are those of the author(s) and do not necessarily reflect the opinions of the referenced sources.
Comments