Automating Injury Severity Assessment Using AI: Revolutionizing Personal Injury Case Management
- Dr Suhail Chughtai FRCS
- Dec 12, 2024
- 3 min read
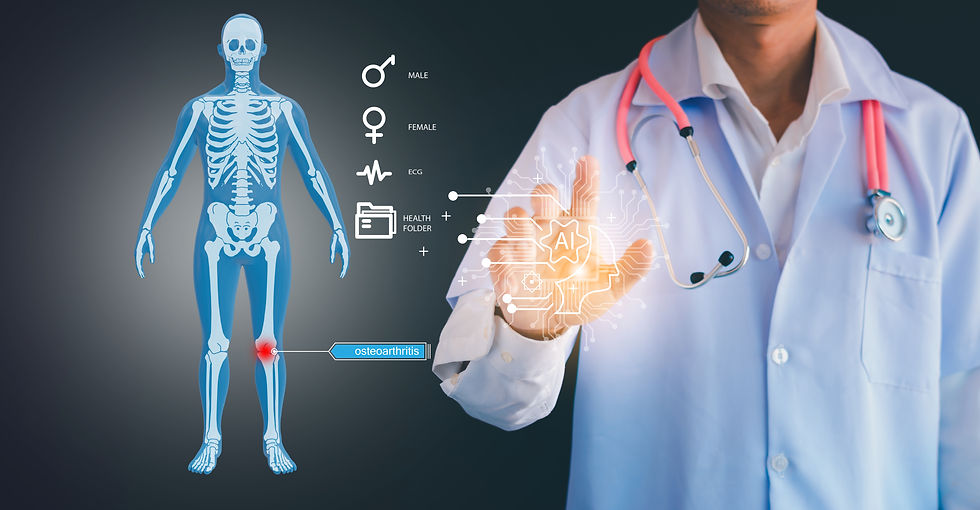
Blog by: Dr. Suhail Chughtai, FRCS, FFLM
Injury severity assessment in personal injury cases requires accurate and standardised analysis of imaging data and clinical evaluations. The integration of AI algorithms into this domain presents a transformative opportunity to enhance objectivity, efficiency, and evidence-based decision-making. This article explores the potential of AI in injury severity assessment, its deployment methodology, associated challenges, and future vision, supported by case studies and research evidence.
Big Data and Evidence-Based Medicine
AI-driven solutions leverage big data to revolutionise evidence-based medicine, optimising workflows and enhancing health outcomes. Machine learning algorithms analyse large datasets from imaging modalities such as X-rays, MRIs, and CT scans to detect patterns correlating with injury severity. This approach ensures a standardised evaluation, minimising variability across different assessors. Big data's role extends to population health management by identifying injury trends and risk factors, guiding public health policies. For example, predictive analytics derived from injury-related imaging and outcomes can inform preventive measures and resource allocation (British Journal of Radiology, 2021). Furthermore, combining imaging data with electronic health records (EHRs) refines predictive capabilities, ensuring more personalised and accurate assessments.
Optimising Hospital Workflows
AI reduces manual workload by automating image segmentation, anomaly detection, and injury grading. Hospitals can benefit from streamlined workflows, wherein radiologists and orthopaedic specialists focus on complex cases while AI handles routine assessments. For instance, algorithms such as convolutional neural networks (CNNs) have demonstrated remarkable accuracy in detecting fractures from X-rays, enabling rapid triage and treatment prioritisation (Radiology: Artificial Intelligence, 2022). By integrating AI into Picture Archiving and Communication Systems (PACS), hospitals ensure seamless analysis and reporting, significantly reducing turnaround times.
IMPROVING PERSONAL INJURY CASE MANAGEMENT
In personal injury litigation, objectivity and precision are paramount. AI enhances fairness by providing standardised injury assessments that eliminate subjective bias. Linking imaging data with clinical findings through natural language processing (NLP) facilitates comprehensive injury profiling, useful for legal and insurance evaluations. For example, deep learning algorithms can quantify soft tissue damage or detect minute structural abnormalities, supporting more accurate injury grading. A notable case study involves the use of AI to analyse MRI scans for whiplash-associated disorders, showing 87% accuracy in correlating radiological findings with clinical outcomes (Journal of Orthopaedic Research, 2023). Such advancements promise greater confidence in medico-legal assessments.
DEPLOYMENT METHODOLOGY
Deploying AI in injury severity assessment involves the following key steps:
Data Aggregation
Collecting and anonymising diverse imaging datasets and clinical records.
Model Training
Using supervised learning models, such as CNNs or recurrent neural networks (RNNs), trained on labeled datasets to recognise injury patterns.
Integration
Embedding AI into clinical workflows, including PACS and EHRs.
Validation
Ensuring AI output matches clinical expertise through validation studies.
Regulatory Compliance
Meeting standards such as the UK’s National Institute for Health and Care Excellence guidelines for AI implementation in healthcare. However, challenges include data privacy concerns, the need for diverse and high-quality datasets, and clinician resistance due to perceived loss of autonomy.
CASE STUDIES SUPPORTING AI ADOPTION
Fracture Detection Models
A study at a UK hospital demonstrated that AI reduced missed fracture rates by 20%, supporting faster patient throughput (Lancet Digital Health, 2022).
AI in Spine Imaging
AI-enabled MRI analysis identified degenerative disc disease with 92% specificity, correlating well with clinical symptomatology (European Spine Journal, 2022). These examples highlight AI's utility in aligning radiological findings with clinical assessments, improving decision-making in both healthcare and legal contexts.
FUTURE VISION
The future of AI in injury severity assessment is promising, driven by advancements in algorithmic precision, cloud-based interoperability, and wearable sensor integration. With continued innovation, AI systems could predict long-term outcomes such as disability risks and rehabilitation needs, aiding personalised recovery plans. Furthermore, federated learning, a decentralised approach to training algorithms across multiple institutions, offers a path to harness diverse datasets while safeguarding privacy. By 2030, AI will likely be a cornerstone of medico-legal practice, enabling rapid and objective injury evaluations that enhance patient care, optimise resources, and uphold justice in personal injury cases (Nature Medicine, 2023).
Conclusion
The integration of AI into injury severity assessment offers unprecedented opportunities for standardisation, efficiency, and fairness. As hospitals and legal entities adopt these technologies, overcoming deployment challenges will be critical. Supported by robust evidence and innovation, AI-driven assessments will redefine injury management, benefiting healthcare systems, legal frameworks, and affected individuals alike.
..........................................................................................................
DISCLAIMER
The content presented in this publication includes references, insights, and excerpts derived from external sources and authors. Every effort has been made to credit the original authors and sources appropriately. If any oversight or misrepresentation is identified, it is unintentional, and we welcome corrections to ensure proper attribution. The inclusion of external materials does not imply endorsement or affiliation with the original authors or publishers. This publication is intended for informational and educational purposes only, and the views expressed are those of the author(s) and do not necessarily reflect the opinions of the referenced sources.
Comments